Harnessing Machine Learning for Optimized Land Use and Zoning
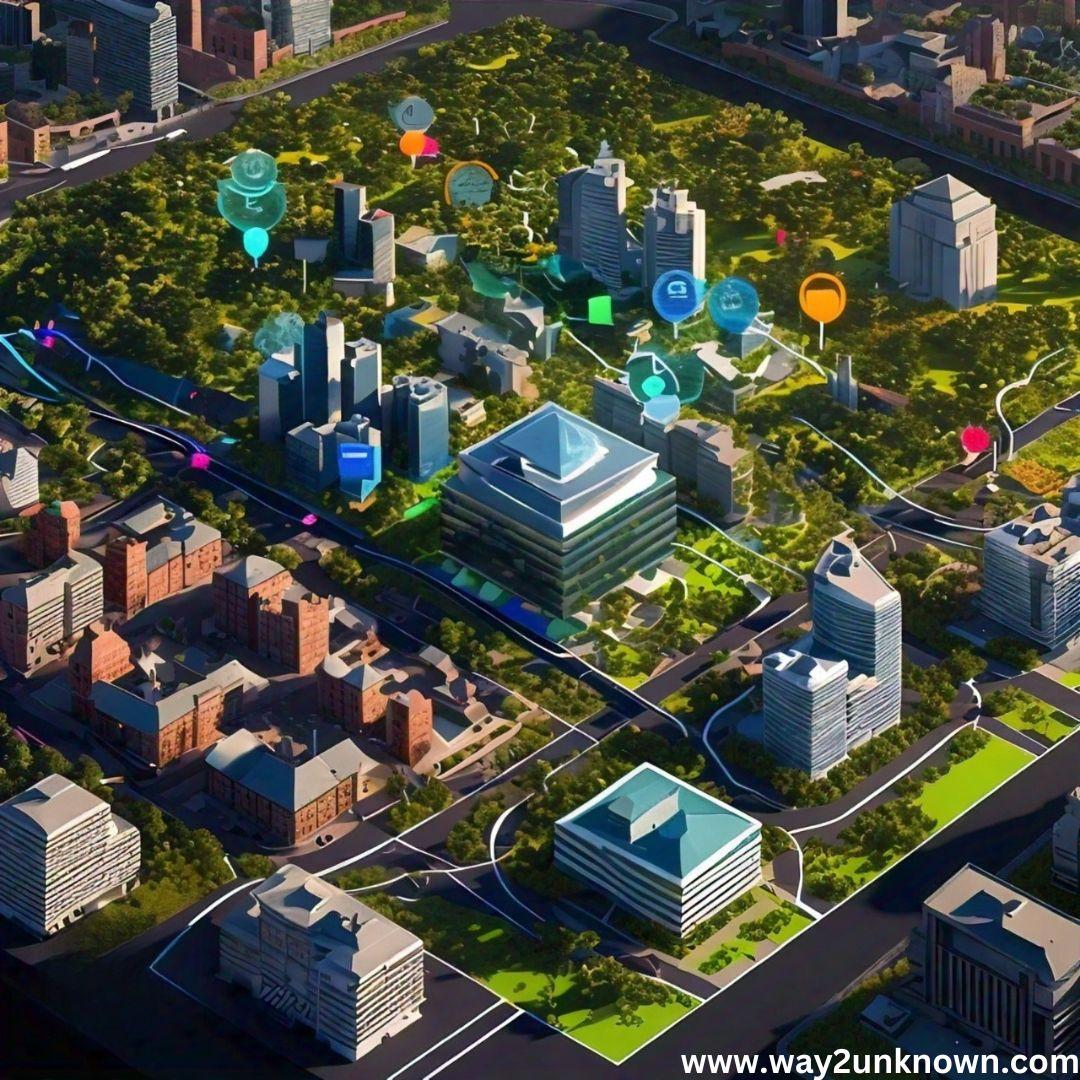
In the realm of urban planning, machine learning (ML) is revolutionizing the approach to land use and zoning by offering sophisticated tools to analyze and predict patterns with remarkable finesse. Traditional methods of land use planning often relied on static models and historical data, which could be limited and slow to adapt to emerging trends. Machine learning, however, leverages vast datasets and advanced algorithms to deliver dynamic insights, enabling planners to make more informed decisions. By analyzing data such as population growth, traffic patterns, and environmental factors, ML algorithms can predict future needs and suggest optimal zoning strategies.
One of the significant advantages of ML in this context is its ability to handle large volumes of complex data. This capability not only enhances the precision of predictions but also allows for more nuanced zoning decisions that consider a wide range of variables. However, the integration of ML in land use planning also raises important considerations related to privacy and bias. As planners collect and utilize data, ensuring that personal information is protected and that the algorithms do not perpetuate existing biases is crucial. Implementing robust privacy measures and continuously monitoring for biases in ML models can mitigate these risks, ensuring that the technology benefits all stakeholders equitably.
By adopting machine learning, cities and municipalities can optimize land use and zoning with unprecedented accuracy, ultimately leading to more sustainable and efficient urban environments. This technology empowers planners to anticipate future challenges and opportunities, transforming traditional approaches and paving the way for smarter, data-driven urban development. As machine learning continues to evolve, its role in urban planning is set to become even more integral, driving progress and innovation in how we design and manage our cities.